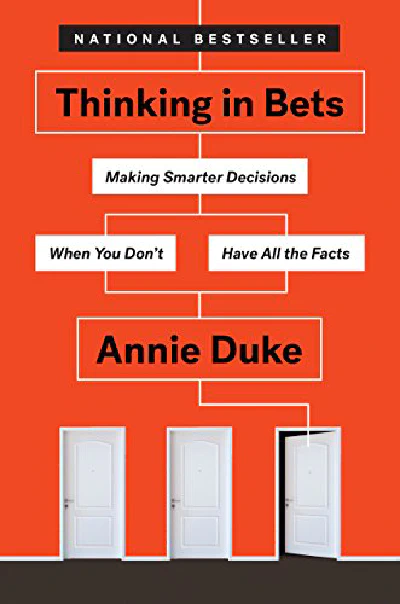
Thinking in Bets - Chapter 6 - Mental Time Travel
Did you know that losing $1000 and then winning $900 (i.e. getting a result of -$100 in total) is more pleasant than winning $1000 and then losing $900 (i.e. getting a result of +$100 in total)!??
What has happened in the RECENT PAST drives our emotional response much more than how we are doing overall! It is a problem if you are making important prediction.
There are to ways to overcome this problem: